Measuring the ROI of Data Annotation: Quantifying the Value of Quality Data
In the realm of artificial intelligence (AI), the adage “garbage in, garbage out” holds particularly true. The quality of data used to train AI models profoundly influences their performance and, consequently, their return on investment (ROI). Data annotation, the process of labeling raw data for machine learning, is a pivotal step in ensuring data quality, but it comes with its costs and challenges. In this article, we explore the intersection of data annotation and ROI, examining how organizations can quantify the value of quality data and make strategic decisions to optimize their AI investments.
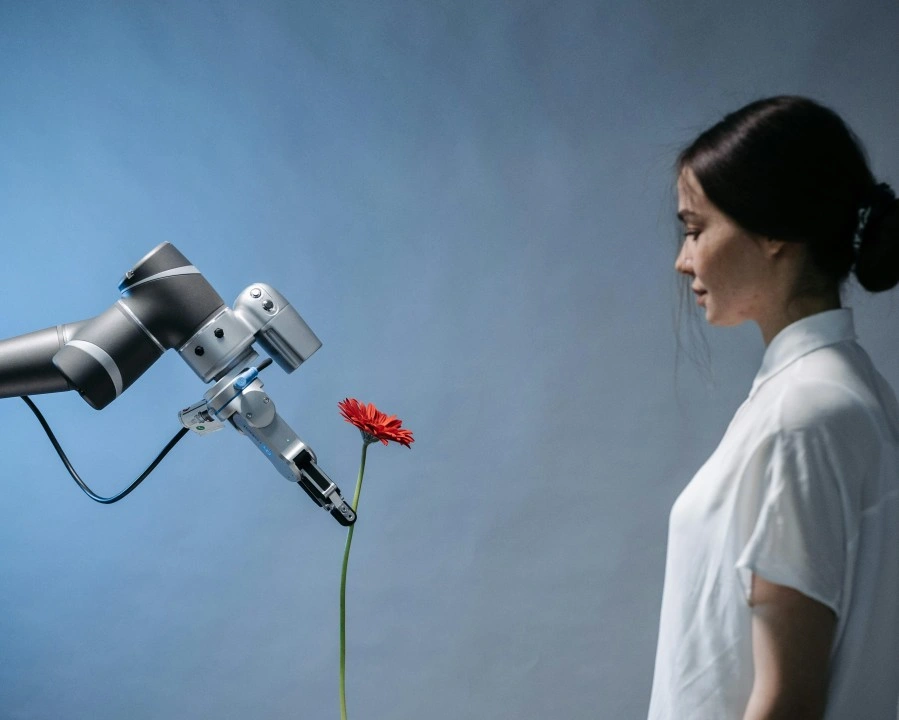
The Foundation of AI: Quality Data
At the core of every successful AI initiative lies a foundation of high-quality, accurately annotated data. Whether it’s image recognition, natural language processing, or predictive analytics, AI algorithms rely on vast datasets to learn patterns and make informed decisions. However, achieving this quality requires meticulous annotation, which can be resource-intensive and time-consuming.
Synaptica.ai: Your Trusted Partner in Data Annotation
At Synaptica.ai, we specialize in delivering tailored data annotation solutions for computer vision applications. We provide the expertise and precision needed to ensure your AI models are fed with the clean, accurate, and contextually relevant data required to perform at their best.
What Sets Us Apart
The Foundation of AI: Quality Data
At the core of every successful AI initiative lies a foundation of high-quality, accurately annotated data. Whether it’s image recognition, natural language processing, or predictive analytics, AI algorithms rely on vast datasets to learn patterns and make informed decisions. However, achieving this quality requires meticulous annotation, which can be resource-intensive and time-consuming.
The Cost of Data Annotation
Data annotation encompasses various approaches, from manual labeling by human annotators to semi-automated tools and crowdsourcing platforms. Each method carries its costs, including labor, software, and quality control measures. While these expenses may seem significant, they pale in comparison to the potential costs of deploying AI models trained on poor-quality data, such as erroneous predictions, reputational damage, or regulatory non-compliance.
Understanding the Value of Data Annotation
Before delving into measuring ROI, it’s essential to understand why data annotation holds such significance. In fields like machine learning, where algorithms learn from labeled data, the quality and quantity of annotations directly impact model performance. Without accurately annotated data, machine learning models can’t effectively generalize patterns or make accurate predictions.
Data annotation not only enhances model accuracy but also reduces the time and resources spent on iterative model training. By providing clear labels and context, annotated data accelerates the learning process, allowing models to achieve satisfactory performance levels with fewer iterations.
Measuring ROI
Calculating the ROI of data annotation involves a structured approach:
Define Metrics: Identify KPIs that measure the impact of AI models on business outcomes, such as revenue, cost savings, or customer satisfaction.
Baseline Assessment: Establish a performance baseline using existing AI models trained on unannotated or low-quality data.
Annotation Investment: Calculate the total cost of data annotation, including labor, software, and associated expenses.
Performance Improvement: Measure the performance gains achieved by AI models trained on high-quality annotated data compared to the baseline.
ROI Calculation: Use the formula: ROI = (Net Benefits / Annotation Costs) x 100% to determine the return on investment.
Quantifying the Benefits
Improved Model Performance: One way to measure the ROI of data annotation is through improved model performance metrics such as accuracy, precision, recall, and F1 score. Comparing the performance of models trained with and without annotated data can provide insights into the impact of annotation efforts.
Time Savings: Another aspect of ROI lies in the time saved during model development and deployment. Efficiently annotated data reduces the time required for training and fine-tuning models. Organizations can calculate the cost savings associated with reduced development cycles and faster time-to-market.
Resource Optimization: Quality data annotation can lead to resource optimization by minimizing the need for manual intervention and post-processing of model outputs. This not only reduces labor costs but also enhances the scalability of machine-learning solutions.
The Future of AI: Built on Better Data
Measuring the ROI of data annotation is essential for organizations seeking to justify their investment in quality data. By quantifying improvements in model performance, time savings, and resource optimization, businesses can demonstrate the tangible benefits of data annotation efforts. As data continues to drive innovation across industries, understanding and optimizing the ROI of data annotation will become increasingly critical for success.