Empowering AI with Precision Annotation Services by Synaptica.ai
At Synaptica.ai, we specialize in delivering precise annotation services essential for high-impact AI applications across industries. Our range of services—including Bounding Box, Polygon, Keypoint, Polyline, and 3D Cuboid annotations—ensures your data is labeled with accuracy and efficiency, laying the groundwork for robust machine learning and computer vision models.
Our services go beyond standard data labeling. We’re dedicated to providing adaptable solutions that cater to unique project requirements, whether you need complex object identification, movement tracking, or feature extraction. With Synaptica.ai’s scalable services, clients can seamlessly manage high-volume projects, ensuring that models are trained with consistently accurate data for reliable performance.
By choosing Synaptica.ai, you gain access to a team of annotation experts, cutting-edge tools, and a commitment to quality that transforms raw data into actionable intelligence. Elevate your AI models with services that are built to support rapid, impactful innovation across every stage of your project.
Image Annotation
Synaptica.ai
Bounding Box Annotation
Bounding box annotation is a method in computer vision where rectangular boxes are drawn around objects in images. This technique helps train machine learning models, especially for object detection, by defining the location, size, and scale of objects. The annotated boxes guide the model to recognize and locate objects in new, unseen images. It’s widely used in tasks like detecting faces, vehicles, and products in various industries.
Examples:
• Detecting pedestrians, cars, and obstacles in autonomous driving systems.
• Managing inventory by tracking items on shelves in retail stores.
• Identifying faces in surveillance cameras for security purposes.
Why it works:
Bounding boxes give machines a clear reference point to start recognizing objects. By training the model to spot objects within these boxes, it can become better at understanding the visual world around it.
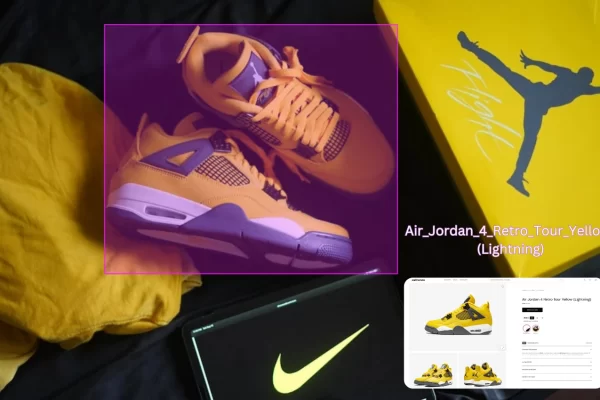
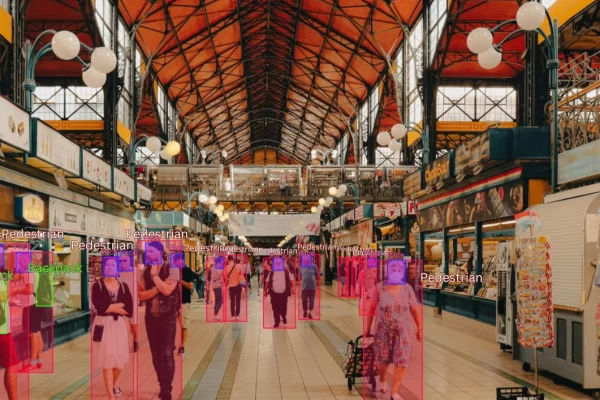
Polyline annotation
Polyline annotation is a computer vision technique used to trace the edges or paths of objects by drawing connected line segments. It’s ideal for labeling linear structures like roads, lanes, pipelines, or boundaries in images. This method captures long, narrow, or continuous shapes accurately. Polyline annotation is commonly used in areas like autonomous driving, mapping, and construction planning.
Examples:
• Detecting road lanes to guide self-driving cars.
• Mapping infrastructure like power lines or road networks in cities.
• Identifying cracks in buildings or bridges for maintenance.
Why it works:
Polylines allow machines to track paths and edges, making it easier for them to navigate and understand boundaries in the real world.
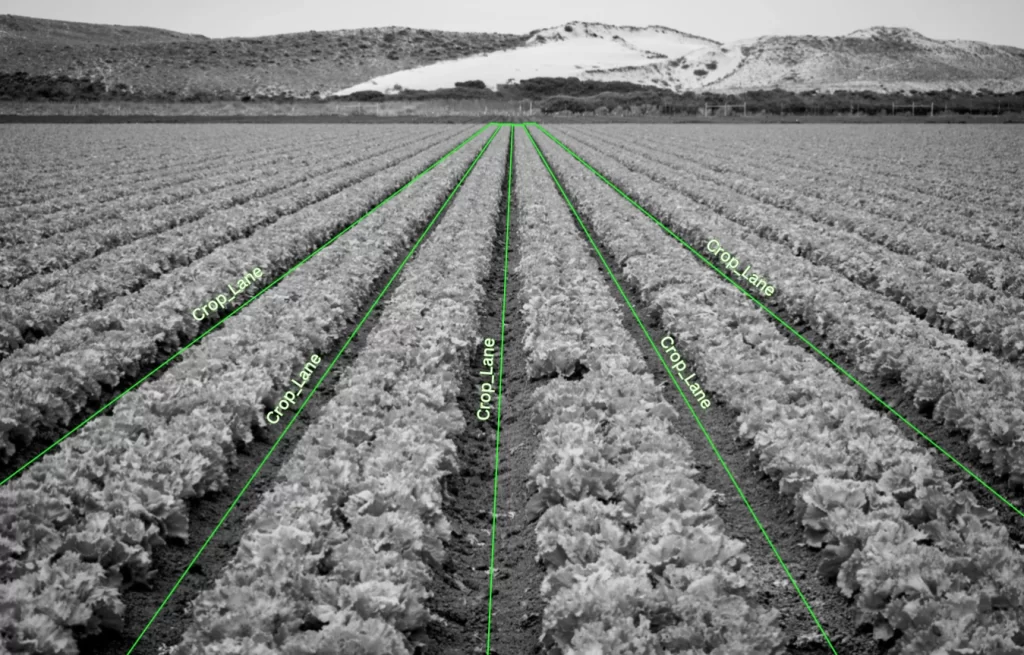
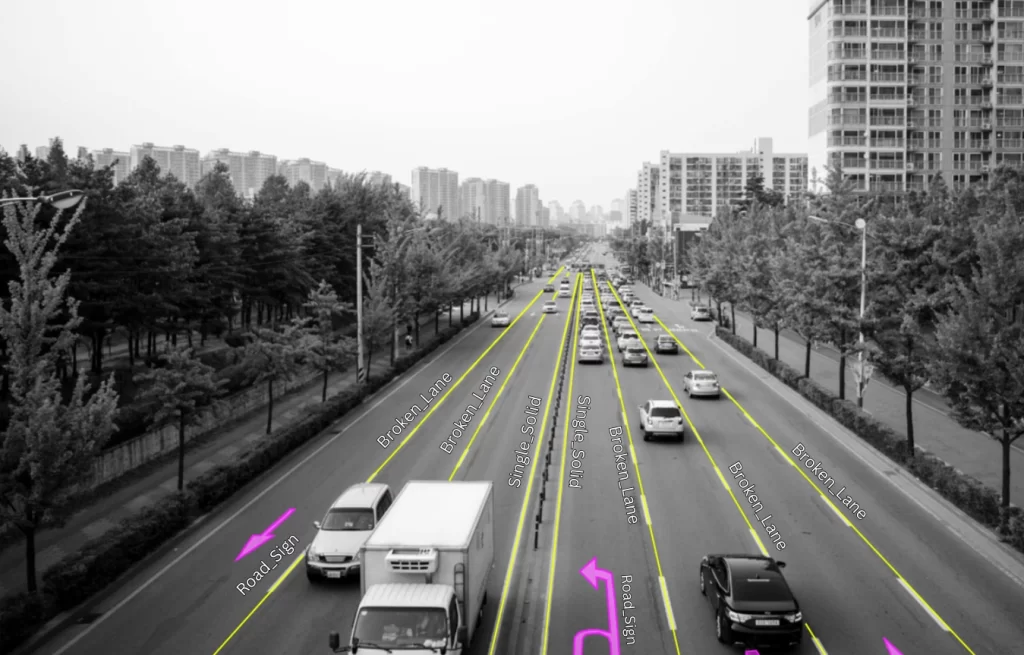
Polygon Annotation
Polygon annotation is a method used to label objects with irregular shapes by marking their exact boundaries using connected points. It offers more precise object detection and segmentation compared to rectangular bounding boxes, as it can fit complex or curved objects better. This technique is ideal for tasks requiring detailed accuracy, such as detecting asymmetrical shapes in images. It is commonly used in fields like autonomous vehicles, medical imaging, and agriculture.
Examples:
• Identifying specific areas in medical images, like tumors in scans.
• Analyzing environmental changes in satellite imagery, such as tracking deforestation.
• Improving obstacle detection for self-driving cars by capturing the exact shape of objects.
Why it works:
With polygons, you give Al more detail about an object’s true shape, leading to better detection and more accurate decisions in complex environments

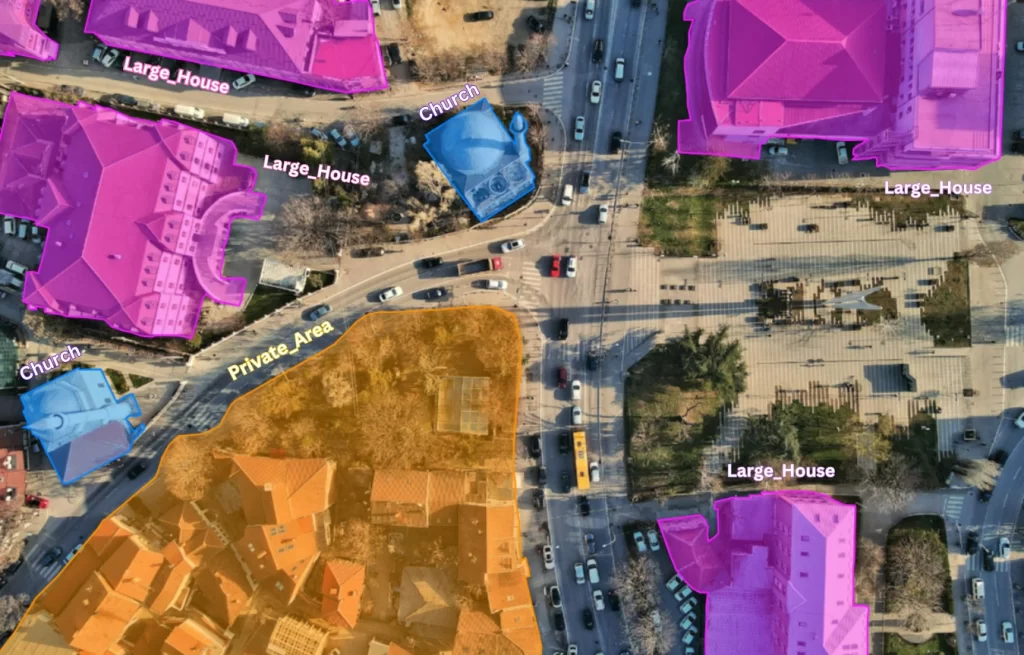
Keypoint Annotation
Keypoint annotation is a method in computer vision that marks specific points of interest on objects, like facial landmarks or body joints. It helps capture the shape, movement, or key features of objects. This technique is useful for tasks such as pose estimation, facial recognition, and action tracking. Each point represents a crucial part of the object, allowing models to understand its structure.
Examples:
• Estimating body movement for fitness apps or rehabilitation.
• Tracking facial features for security systems or user identification.
• Analyzing player movements in sports to enhance performance.
Why it works:
By focusing on key points, Al models learn to recognize patterns and track movements more efficiently, which is especially valuable for systems analyzing human actions.
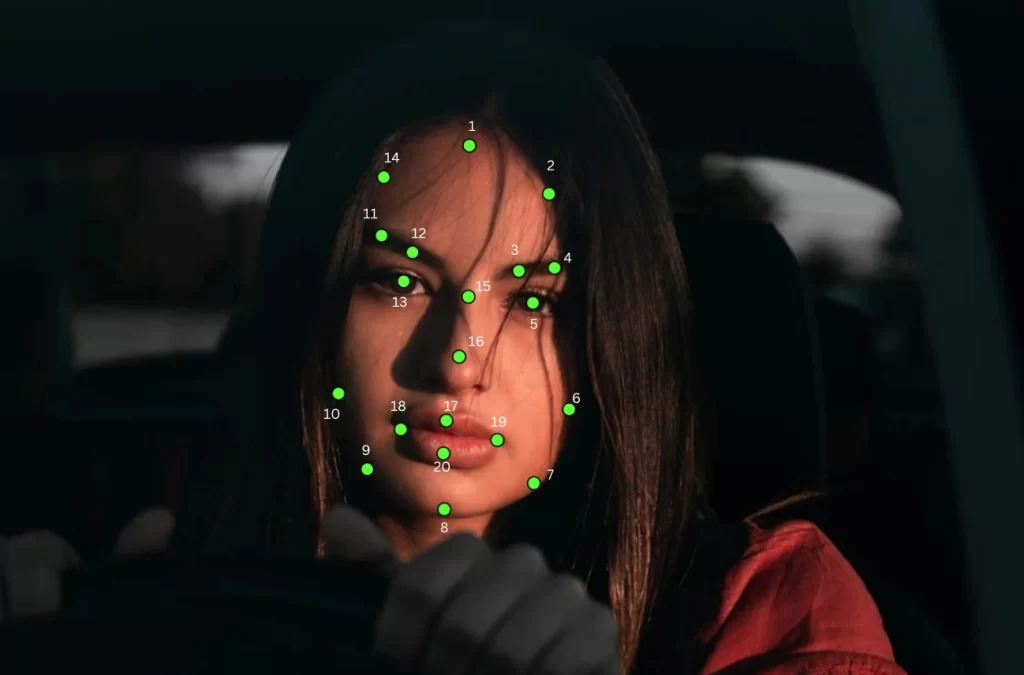
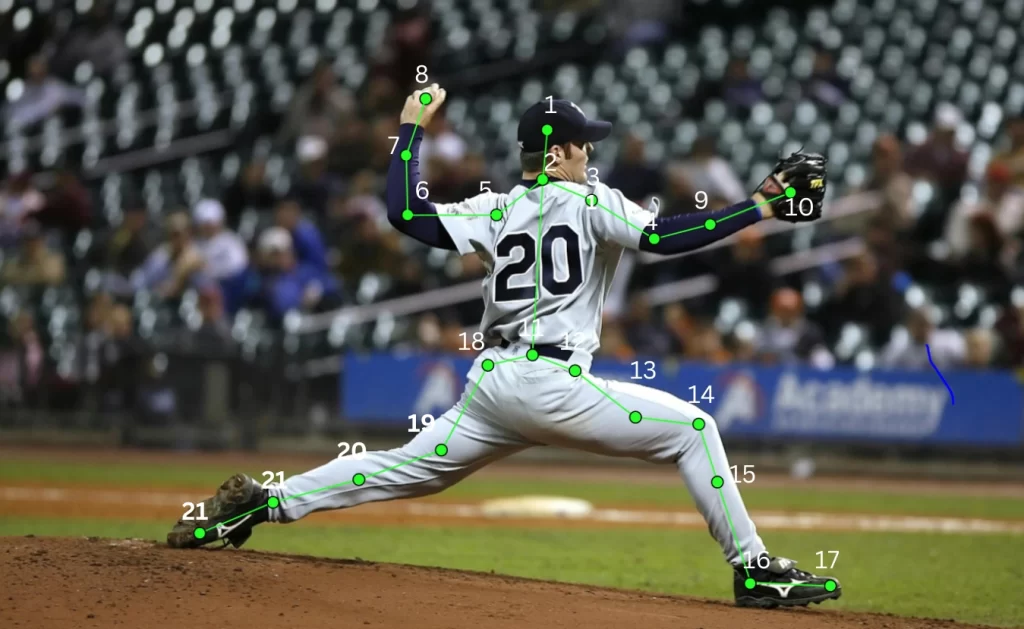
3D Cuboid Annotation
3D Cuboid Annotation is a computer vision technique that involves drawing three-dimensional boxes around objects to provide depth information. This method captures the depth, width, and height of objects, offering a more accurate representation of their size and position in space compared to 2D bounding boxes. It’s particularly useful for applications like autonomous driving, where understanding the 3D position of vehicles and obstacles is crucial.Thistechnique enhances models’ local awareness and depth perception.
Examples:
• Identifying vehicles, pedestrians, or obstacles in 3D for self-driving cars.
• Helping robots grasp and manipulate objects in warehouses.
• Managing inventory in automated warehouses by understanding object size and location.
Why it works:
Cuboids help Al systems work in three dimensions, giving them a fuller understanding of their environment. This makes them more reliable when interacting with objects in the real world.
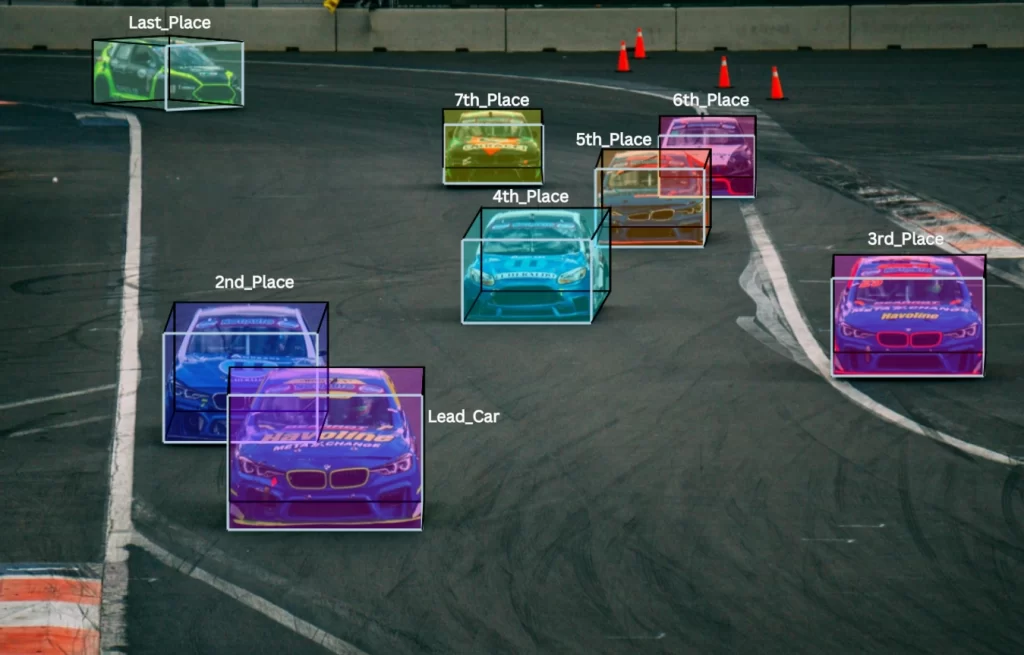
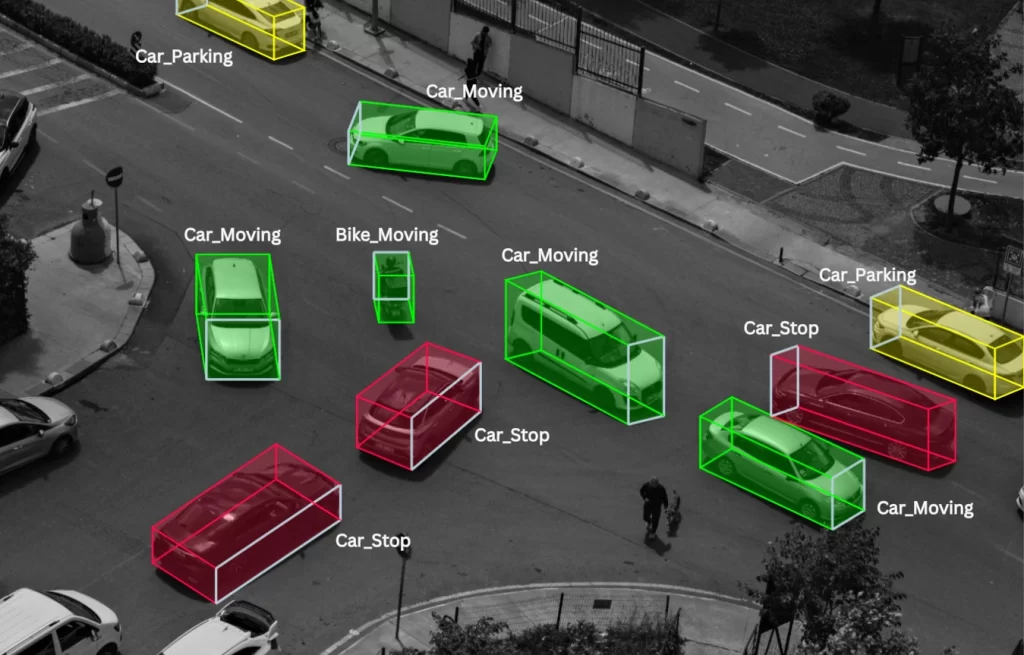
Video
Annotation
Synaptica.ai
Video Annotation
Labels objects, actions, or events in video frames for AI recognition. Enhances object tracking and scene understanding across sequences.
- Autonomous Vehicles: Track vehicles, pedestrians.
- Sports Analytics: Analyze player movements.
- Surveillance: Monitor activity.
Why it works:
Helps AI understand motion and context for accurate decisions.
Text Annotation
Synaptica.ai
Text Classification
Text classification is a type of text annotation where text data is labeled into predefined categories based on its content. This technique helps AI models organize, analyze, and understand text by assigning it to the most relevant category, making it useful for large-scale content management and analysis.
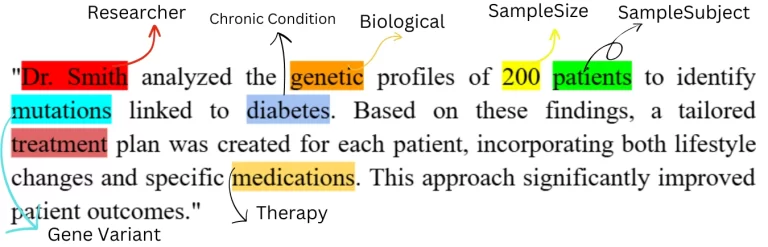
Examples:
• Categorizing customer support tickets into topics like billing, technical issues, or account inquiries.
• Labeling news articles into sections such as sports, politics, entertainment, or business.
• Classifying spam versus non-spam emails to improve email filtering systems.
Why it works:
Text classification simplifies the process of organizing large volumes of data by assigning clear and consistent labels. This annotation enhances an AI model’s ability to identify patterns, improve content tagging, and deliver relevant insights, boosting efficiency in applications like content recommendation, sentiment analysis, and automated moderation.
Named Entity Recognition (NER)
Named Entity Recognition (NER) is a type of text annotation used to identify and label specific entities within text, such as names, locations, organizations, dates, or other predefined categories. This method helps AI models understand structured information within unstructured text data, making it essential for applications requiring data extraction or categorization.
Examples:
• Extracting company names, dates, and monetary values from legal documents.
• Identifying person names, locations, and events in news articles.
• Highlighting product names and specifications in customer feedback for e-commerce platforms.
Why it works:
NER provides a structured way to convert raw text into meaningful data by marking key entities. This detailed labeling improves the AI’s ability to extract and analyze specific information, enabling more efficient document management, personalized content delivery, and data-driven decision-making across industries.
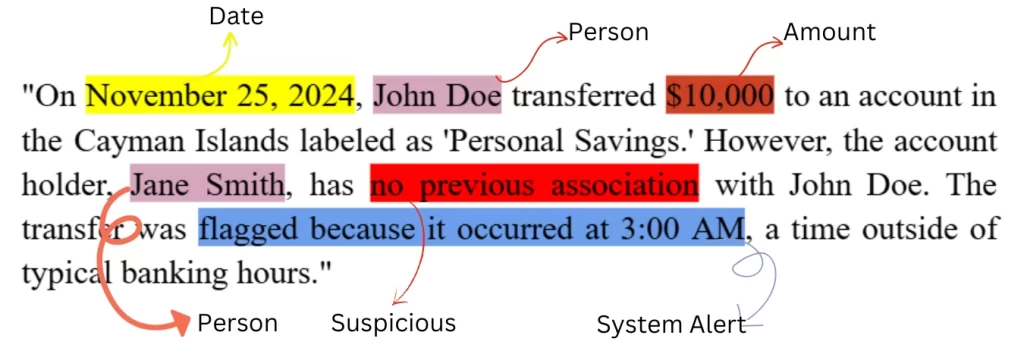
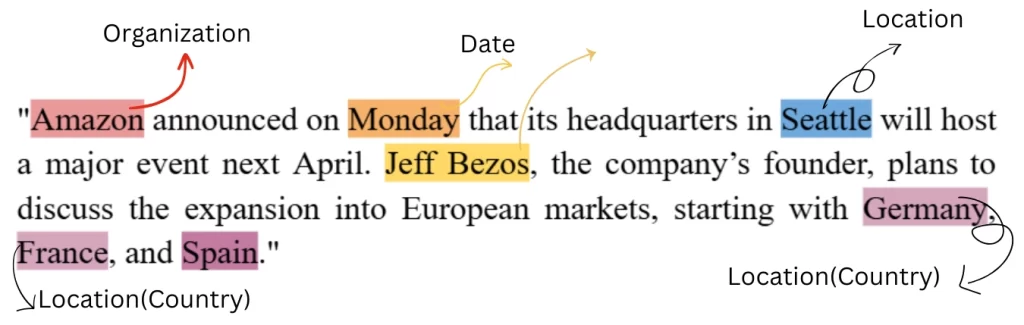
Sentiment Analysis
Labels text to classify emotions, opinions, or attitudes, helping AI understand tone and context.
- Customer Feedback: Identify positive, neutral, or negative reviews.
- Social Media: Monitor sentiment during events or crises.
- E-commerce: Detect satisfied vs. dissatisfied users.
Why it works:
Captures emotional cues, linguistic nuances, and context for accurate sentiment detection, improving AI-driven insights.
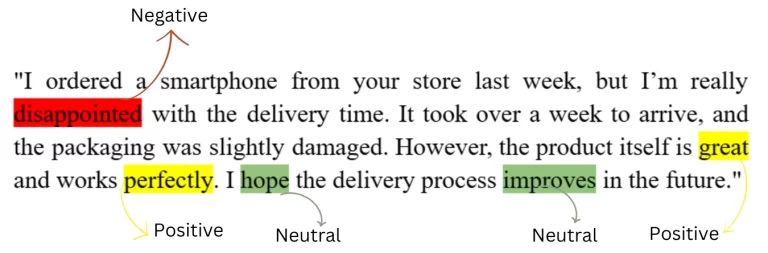
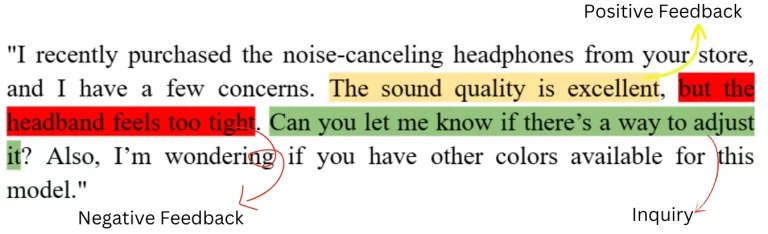