The Evolving Role of Data Annotation: Will Automation Eclipse Human Expertise?
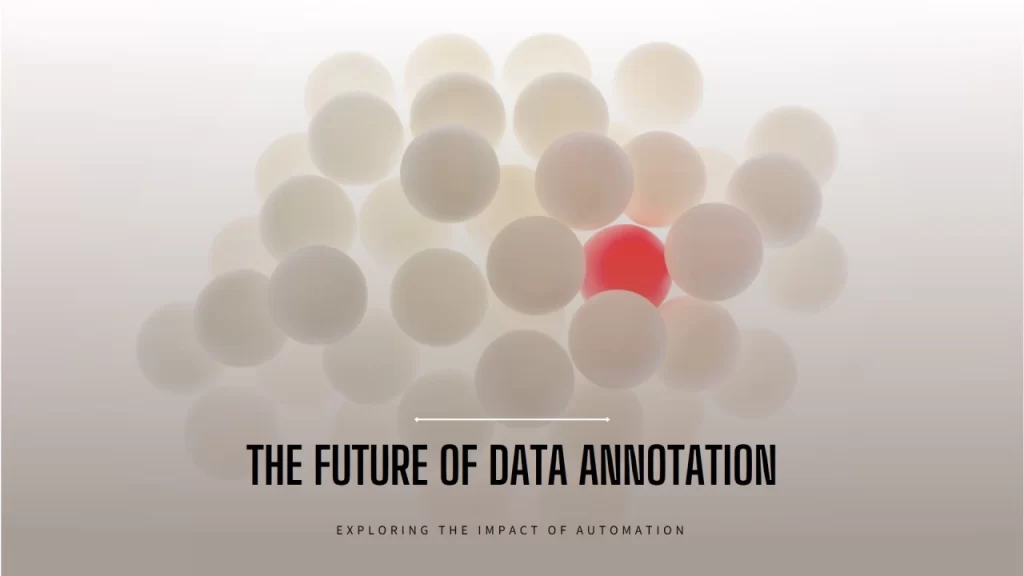
Data annotation has long been a cornerstone of developing accurate and reliable models in the ever-evolving landscape of artificial intelligence (AI) and machine learning (ML). Traditionally a labor-intensive process, it has required meticulous human effort to tag, label, and categorize vast amounts of data. However, with the advent of advanced annotation tools and automation technologies, many are questioning the future of data annotation. Will the skill of data annotation become obsolete? Or will the industry adapt and thrive in this new era?
The Rise of Advanced Annotation Tools
Modern annotation tools have revolutionized the way data is tagged and processed. These tools leverage sophisticated algorithms and user-friendly interfaces to facilitate faster and more efficient data labeling. Features such as semi-automated tagging, predictive text, and smart data integration have drastically reduced the manual effort required. For instance, tools equipped with machine learning capabilities can now suggest labels based on previous annotations, significantly speeding up the process.
Synaptica.ai: Your Trusted Partner in Data Annotation
At Synaptica.ai, we specialize in delivering tailored data annotation solutions for computer vision applications. We provide the expertise and precision needed to ensure your AI models are fed with the clean, accurate, and contextually relevant data required to perform at their best.
What Sets Us Apart
The Automation Trend
Automation is the most significant shift in the data annotation field. Machine learning models can now automate routine annotation tasks, such as object detection in images or sentiment analysis in text. This not only accelerates the annotation process but also enhances consistency and reduces human error. As automation becomes more prevalent, there is a growing concern that the role of human data annotators might diminish, leading to potential job losses and a decline in the industry.
The Human Element in Data Annotation
Despite the advancements in automation, the role of human annotators remains crucial. Automated tools and algorithms, while powerful, still lack the nuanced understanding that humans bring to the table. Human annotators can grasp context, resolve ambiguities, and apply domain-specific knowledge that machines often struggle with. For example, annotating complex medical images or understanding cultural nuances in language requires a level of insight that current AI systems cannot fully replicate.
Furthermore, the development and maintenance of automated annotation tools themselves rely on human expertise. Data scientists and engineers must continuously refine these tools, ensuring they are accurate and effective. This iterative process requires a deep understanding of both the data and the algorithms used for annotation.
The Future of Data Annotation
Rather than seeing data annotation as a field in decline, it is more accurate to view it as an industry in transformation. As automation takes over routine tasks, the demand for human annotators is shifting towards more complex and specialized areas. Annotators will increasingly focus on tasks that require critical thinking, contextual understanding, and domain expertise.
Additionally, the rise of automation opens new opportunities for data annotators to work alongside advanced tools, enhancing their productivity and expanding their roles. Training and upskilling will be essential for annotators to stay relevant in this evolving landscape. By embracing new technologies and adapting to changing demands, data annotators can continue to play a vital role in the AI and ML ecosystems.
The Future of AI: Built on Better Data
While automation and advanced annotation tools are reshaping the data annotation industry, they are not heralding its end. Instead, they are transforming it, creating new opportunities, and redefining the skills required. The integration of automation should be seen as a complementary force, augmenting human expertise rather than replacing it. As long as there are complex and context-sensitive tasks, the need for skilled data annotators will persist. The industry will continue to thrive by adapting to these changes and leveraging both human and machine strengths.